Reviewers, and even associate editors, sometimes criticize studies for being “overpowered” – that is, for having sample sizes that are too large. (Recently, the between-subjects sample sizes under attack were about 50-60 per cell, just a little larger than you need to have an 80% chance to detect that men weigh more than women).
This criticism never makes sense.
The rationale for it is something like this: “With such large sample sizes, even trivial effect sizes will be significant. Thus, the effect must be trivial (and we don’t care about trivial effect sizes).”
But if this is the rationale, then the criticism is ultimately targeting the effect size rather than the sample size. A person concerned that an effect “might” be trivial because it is significant with a large sample can simply compute the effect size, and then judge whether it is trivial.
(As an aside: Assume you want an 80% chance to detect a between-subjects effect. You need about 6,000 per cell for a "trivial" effect, say d=.05, and still about 250 per cell for a meaningful "small" effect, say d=.25. We don't need to worry that studies with 60 per cell will make trivial effects be significant).
It is OK to criticize a study for having a small effect size. But it is not OK to criticize a study for having a large sample size. This is because sample sizes do not change effect sizes. If I were to study the effect of gender on weight with 40 people or with 400 people, I would, on average, estimate the same effect size (d ~= .59). Collecting 360 additional observations does not decrease my effect size (though, happily, it does increase the precision of my effect size estimate, and that increased precision better enables me to tell whether an effect size is in fact trivial).
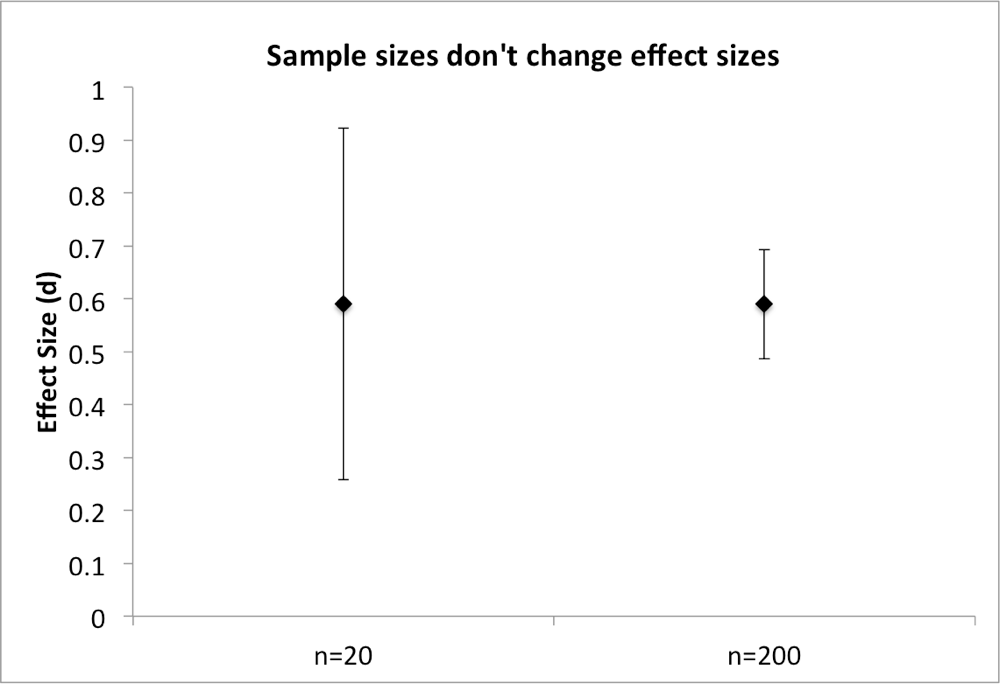
Our field suffers from a problem of underpowering. When we underpower our studies, we either suffer the consequences of a large file drawer of failed studies (bad for us) or we are motivated to p-hack in order to find something to be significant (bad for the field). Those who criticize studies for being overpowered are using a nonsensical argument to reinforce exactly the wrong methodological norms.
If someone wants to criticize trivial effect sizes, they can compute them and, if they are trivial, criticize them. But they should never criticize samples for being too large.
We are an empirical science. We collect data, and use those data to learn about the world. For an empirical science, large samples are good. It is never worse to have more data.